FDA guiding for AI in healthcare: good machine learning practices
Artificial intelligence and machine learning are revolutionising healthcare by driving advancements in diagnostics, treatment personalisation, as well as patient outcomes. However, as these technologies rapidly evolve, ensuring their safety, effectiveness, and quality presents a critical challenge. To address this issue, the U.S. Food and Drug Administration, along with Health Canada and the United Kingdom’s Medicines and Healthcare products Regulatory Agency (MHRA), released a joint document in October 2021. The document outlines 10 Guiding Principles for Good Machine Learning Practice (GMLP) in Medical Device Development. Understanding the FDA guiding for AI in healthcare aids medical device and medical software development.
Understanding the FDA guiding principles for AI in healthcare
These principles provide a framework for navigating the complexities of AI/ML-driven medical devices. Moreover, they address challenges such as continuous learning, data reliance, and regulatory alignment.
In this post, we will explore these guiding principles. Moreover, their impact and significance for the future of AI-powered medical devices. Whether you are a developer, healthcare provider, or industry leader, understanding GMLP is essential. Staying informed will help you keep pace with the evolving landscape of AI in medicine. Let’s start.
Why did the FDA develop this guidance?
As AI and machine learning continue to transform the future of medical technology, regulatory frameworks need to evolve accordingly. The FDA, in collaboration with its international partners, has developed guiding principles to establish best practices that ensure the safety and also effectiveness of AI/ML-driven medical devices.
The objective is to adopt proven methodologies from other industries and adapt them to the unique challenges of healthcare. New, sector-specific practices should be created to address the complexities of AI-powered medical tools.
By promoting strong international collaboration, the FDA aims to establish a foundation for responsible innovation. This ensures that regulatory standards keep pace with advances in AI technology while protecting patients.
This initiative supports global efforts. Regulators are working with the International Medical Device Regulators Forum (IMDRF) to promote consistency and harmonization in the regulation of AI and ML medical devices.
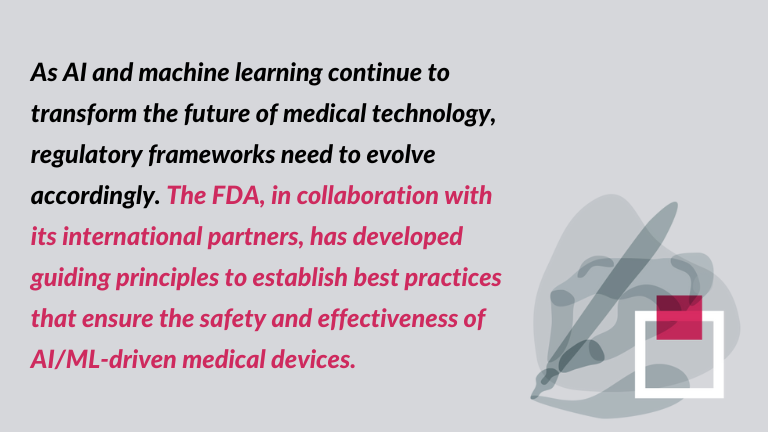
The essential principles for reliable machine learning in healthcare
The 10 Guiding Principles for Good Machine Learning Practice (GMLP) provide a foundation for ensuring that AI-powered medical devices are safe, effective, and of high quality. These principles address the unique challenges posed by AI and machine learning in healthcare while promoting responsible and ethical innovation.
1. Leveraging the multi-disciplinary expertise throughout the total product life cycle:
A thorough understanding of how an AI model integrates into clinical workflows, as well as its potential benefits and risks for patients, is crucial for ensuring the safety and effectiveness of machine learning-based medical devices. Drawing on expertise from various disciplines throughout the product’s lifecycle helps address significant clinical needs and uphold high-performance standards.
2. Implementation of the good software engineering and security practices:
The development of AI models must follow basic software engineering principles. This includes managing high-quality data, implementing strong cybersecurity measures, and conducting thorough risk assessments. A structured approach to design as well as risk management ensures transparency in decision-making while preserving data integrity.
3. Clinical study participants and data sets are representative of the intended patient population:
Clinical studies and datasets ought to faithfully reflect the traits of the intended patient demographic, encompassing elements like age, gender, race, and also ethnicity. Thorough data gathering is crucial to guarantee that AI models operate effectively across various populations. This approach helps reduce bias, improves usability, and uncovers possible constraints in practical applications.
4. Training data sets are independent of test sets:
To guarantee an impartial assessment of performance, it is essential that the training and test datasets are entirely distinct from one another. Aspects like patient overlap, methods of data collection, and influences specific to different sites need to be meticulously controlled to avoid any unintentional dependencies that could undermine the model’s reliability.
5. Selected reference datasets are based on best available methods:
Reference datasets should be created using the most reliable and widely accepted methods to ensure that the data is clinically relevant and well-characterized. Whenever possible, utilizing established reference datasets during model development and testing helps demonstrate the model’s robustness and its ability to generalize to the target patient population.
6. Tailoring the model design to the available data and reflecting the intended use of the device:
The model’s design is carefully crafted to align with the available data. It also addresses risks such as overfitting, performance decline, and security vulnerabilities.
A clear understanding of the product’s clinical benefits and risks helps set meaningful performance goals for testing. This ensures the device can safely and effectively fulfill its intended purpose.
The design process considers several factors. These include global and local performance, variability in inputs and outputs, diversity within the patient population, and the conditions under which the device will be used clinically.
7. Focus is placed on the performance of the human-AI team:
When human collaboration is part of the model, the emphasis shifts to the collective performance of the Human-AI team instead of solely on the model itself. This requires taking into account human elements and even making certain that the outputs of the model are easily understandable by people to improve the partnership between human and AI components.
8. Testing demonstrates device performance during clinically relevant conditions:
Experts carefully create and execute statistically sound testing plans to assess the device’s performance in practical clinical environments, distinct from the training dataset. These evaluations take into account multiple factors, such as the intended patient demographic, important subgroups, the clinical setting, interactions between humans and AI, measurement inputs, and any potential confounding factors.
9. Users are provided with clear, essential information:
Individuals should have easy access to clear and relevant information that meets their needs, whether they are healthcare professionals or patients.
This information should include details about the product’s intended use and its effectiveness across different demographics. It should also cover the type of data used for training and testing, acceptable input formats, and known limitations. Additionally, users should receive guidance on understanding the user interface and how the model integrates into clinical procedures.
Furthermore, users should be informed about any updates or improvements based on real-world performance. They should also understand the reasoning behind decisions when applicable and know how to raise concerns with the developer.
10. Deployed models are monitored for performance and also re-training risks are managed:
After deployment, it is essential to continuously oversee models in practical environments. This ensures that they maintain or improve their safety and performance levels. When models are subject to regular or ongoing retraining, suitable measures need to be implemented to reduce risks like overfitting, inadvertent bias, or declines in performance (for example, dataset drift). These elements can impact the model’s safety and efficiency within the operations of the Human-AI team.
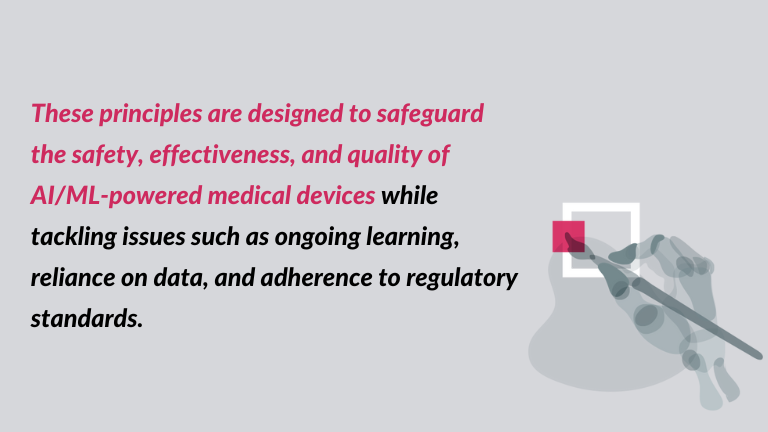
An examination of the FDA’s principles for medical device development
This blog post explores the Guiding Principles for Good Machine Learning Practice (GMLP) in the creation of medical devices. The FDA published these principles in partnership with Health Canada and the UK’s MHRA.
What’s more, the principles may safeguard the safety, effectiveness, and quality of AI/ML-powered medical devices. They also address issues such as ongoing learning, reliance on data, and adherence to regulatory standards.
FDA guiding principles for AI in healthcare: key considerations
We examined how FDA guiding for AI in healthcare establish a framework for responsible innovation. They highlight the significance of multidisciplinary knowledge, sound software engineering practices, as well as the gathering of representative data. Furthermore, the piece emphasizes the necessity of transparent communication with users, continuous monitoring of operational models, and assessing the performance of human-AI collaboration. For developers, healthcare practitioners, and industry stakeholders, grasping and implementing these principles is vital to keep pace with the swiftly changing AI landscape in healthcare.
References
1. Good Machine Learning Practice for Medical Device Development: Guiding Principles, FDA, https://www.fda.gov/medical-devices/software-medical-device-samd/good-machine-learning-practice-medical-device-development-guiding-principles